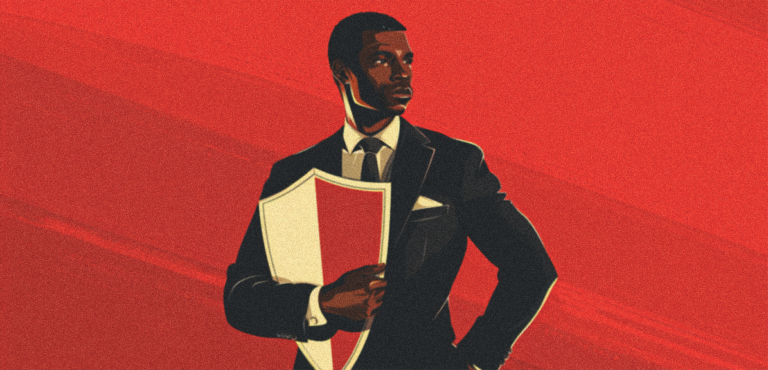
In Conversation: Realities of AI in Financial Services
In an age where data drives decision-making and innovation, the financial sector is undergoing a remarkable transformation. At the forefront of the evolving financial landscape, Motilal Oswal Financial Services, a prominent player in the Indian financial sector has carved out a reputation for its innovative technology backed services. It offers a wide array of services including stock broking, wealth management, and investment banking. Since its inception in 1987, the organization has grown to become synonymous with data-driven financial solutions, consistently leveraging technology to enhance customer experiences and streamline operations.
Heading this digital transformation is Kapil Chandel, Executive Vice President and Head of Data Science at Motilal Oswal Financial Services. In this exclusive interview, Chandel shared his journey, the challenges he faces, and his vision for the future of data science in finance.
Q: Can you share your journey into the field of data science?
Chandel: My journey into data science began over 12 years ago, rooted in my passion for mathematics. After nearly a decade in the IT sector, primarily focused on finance, I recognized the importance of data-driven decision-making. The financial markets are rich with data, and I saw an opportunity to leverage mathematical and statistical techniques to extract actionable insights.
What initially captivated me was the intersection of three critical areas: mathematics, programming, and domain expertise. Transitioning from IT management to analytics roles allowed me to apply my skills in meaningful ways. I started with simple predictive models and gradually advanced to more sophisticated applications, such as machine learning algorithms for portfolio optimization. Today, as the Head of Data Science at Motilal Oswal, I lead a team that employs cutting-edge technologies, including AI and generative AI, to enhance client engagement with financial data.
Q: As a leader in data science, what is your philosophy regarding team dynamics and fostering innovation?
Chandel: Collaboration and autonomy are central to my leadership philosophy. Data science is inherently interdisciplinary, requiring diverse expertise. I foster an environment where team members can freely exchange ideas, as innovation often emerges at the crossroads of different perspectives.
At Motilal Oswal, we prioritize building a culture of continuous learning. Given the rapid evolution of data science, it’s essential for our team to stay updated on trends and technologies. We encourage experimentation and view failure as a vital part of the learning process. This approach has enabled us to achieve breakthrough solutions that drive real business value.
Q: What do you see as the primary limitations of current deep learning approaches, and how might these be addressed in future developments?
Chandel: The primary limitations of current deep learning approaches include their heavy reliance on large, labeled datasets, lack of interpretability, high computational demands, and challenges with generalization and robustness. Moreover, these models can perpetuate biases present in training data.
To address these limitations, future developments will likely focus on data-efficient learning methods, such as unsupervised and transfer learning, which can reduce the need for large, labeled datasets. Enhancing explainability through explainable AI techniques will also be crucial, allowing stakeholders to understand how models make decisions. Additionally, improving efficiency with model compression and edge AI will help mitigate computational demands. Finally, ensuring fairness by incorporating bias detection and ethical frameworks into model development will be vital in promoting responsible AI practices.
Q: With the increasing focus on data privacy, how do you approach ethical considerations in your work?
Chandel: Ethical considerations are integral to our data science initiatives at Motilal Oswal. I believe in adopting a “privacy by design” approach, integrating privacy measures into every stage of AI/ML development. Techniques like differential privacy and federated learning are crucial in protecting sensitive data while ensuring robust model performance.
For instance, we utilize differential privacy to add noise to our datasets, which allows us to derive insights without exposing individual data points. This method helps maintain the utility of our models while safeguarding customer privacy. Furthermore, we prioritize compliance with data protection regulations and actively work to detect and mitigate biases within our models, ensuring fairness and ethical use of AI.
Q: How do you involve stakeholders in discussions about privacy and AI/ML technology to ensure their concerns are addressed?
Chandel: Involving stakeholders in discussions about privacy and AI/ML technology is critical, particularly in the financial services industry, where data privacy and ethical AI usage are paramount. My approach emphasizes transparency, education, and collaboration.
Transparency is essential. When working with AI/ML models that utilize sensitive customer data, it’s crucial to clearly communicate what data is being used, how it’s processed, and the purpose of the analysis. I ensure that stakeholders—including business leaders, legal teams, and compliance officers—understand the entire lifecycle of an AI/ML project, fostering trust and allowing them to voice their concerns early in the process.
Education also plays a significant role. AI and machine learning can often seem like black boxes to non-technical stakeholders, which can lead to unease regarding privacy and ethical use. I take the time to explain the underlying technologies in simple terms and discuss how we mitigate risks, using techniques like anonymization and differential privacy. This helps stakeholders feel more confident in the technologies we employ.
Collaboration is vital; I actively involve stakeholders in key stages of projects. Before deploying any AI/ML solution, we hold workshops to review ethical implications, privacy safeguards, and potential biases in the model. Working closely with legal and compliance teams ensures our models provide business value while adhering to regulatory standards.
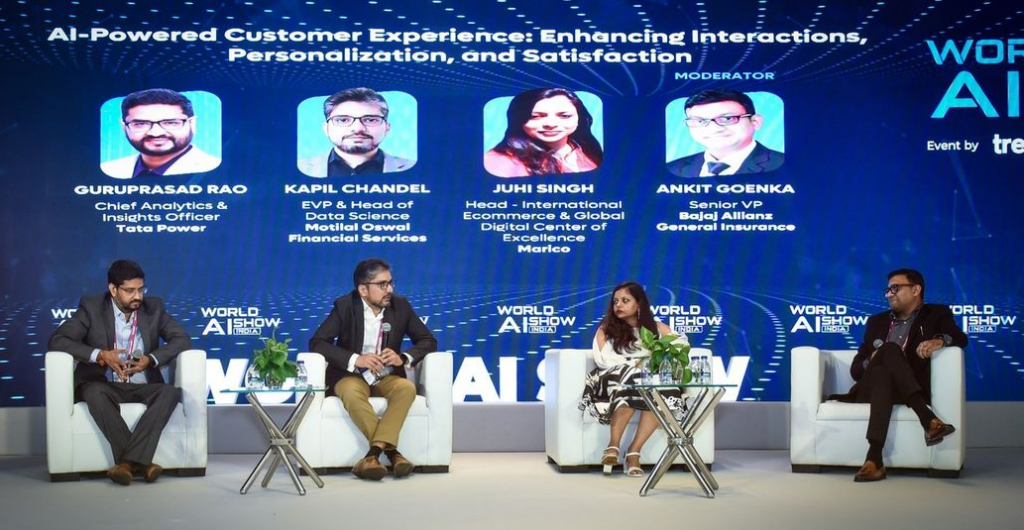
[Mr. Chandel as a panelist at the 43rd World AI Show, organized by Trescon]
Q: How do you envision the relationship between AI/ML technologies and consumer privacy evolving in the next decade?
Chandel: In the next decade, I foresee an increasingly critical relationship between AI/ML technologies and consumer privacy. As data usage proliferates across industries, there will be a growing emphasis on privacy-enhancing technologies as integral components of AI model development. Consumers are becoming more aware of how their data is used, leading to a rising demand for solutions that balance innovation with privacy protection.
Emerging technologies like federated learning will play a pivotal role in this evolution. By allowing AI models to be trained on decentralized data—keeping sensitive data on local devices—federated learning enhances privacy while enabling robust model training. Additionally, differential privacy will ensure that individual data points remain anonymous, allowing organizations to analyze aggregated datasets without compromising user identity.
As AI becomes more embedded in everyday applications, these technologies will become standard practices, enabling organizations to harness powerful models without eroding consumer trust. This shift will not only protect data but also foster a culture of ethical AI development, ensuring that privacy and innovation go hand in hand.
Q: What are some of the biggest challenges you’ve faced in your role, and how have you overcome them?
Chandel: One of the significant challenges I’ve encountered as Head of Data Science has been scaling data-driven solutions across the organization. Motilal Oswal handles vast amounts of data, and while this data is an invaluable asset, integrating it across various business functions and ensuring consistency has been a major hurdle. Early on, we faced issues with data silos, where different teams had their own systems and formats, making it difficult to develop cohesive AI/ML models applicable across the board.
To overcome this, we initiated the development of a centralized data infrastructure that serves as a single source of truth. This involved substantial collaboration between IT, data engineering, and business teams to aggregate and clean data efficiently. We also established robust data governance practices to maintain quality and integrity across the organization.
Another challenge has been managing expectations surrounding AI and machine learning. There is often a misconception that AI can provide instantaneous solutions. However, implementing AI solutions requires careful planning, experimentation, and refinement. I’ve learned the importance of setting realistic expectations with stakeholders—clearly communicating the complexities of the models, the time needed for training and testing, and the limitations of the technology. This approach has helped bridge the gap between excitement and practical application.
Lastly, attracting and retaining top talent in data science has been a challenge, particularly in a competitive field. To address this, I’ve focused on cultivating a culture of continuous learning and growth within our team. We encourage experimentation, provide upskilling opportunities, and allow team members to work on cutting-edge projects. By investing in our people, we’ve built a strong, motivated team that feels empowered to tackle complex challenges.
Explore more of the AI in the industry series.