Article
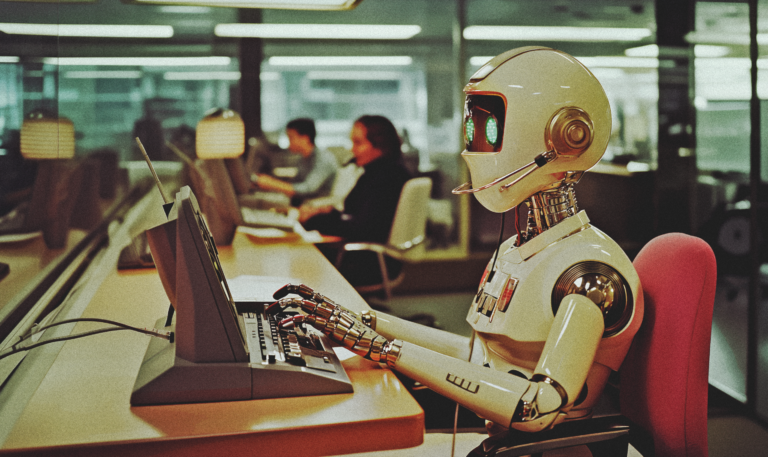
Agentic AI Breaks Through Traditional GenAI Models
The buzz around Agentic AI represents a charting trend in AI development that centers on the capacity to function independently, learn from mistakes, and adjust to novel circumstances without human assistance.
Autonomous systems possess the ability to operate with minimal human intervention, making them particularly suited for tasks that require continuous monitoring or rapid decision-making. They exhibit advanced problem-solving capabilities, enabling them to address complex challenges in innovative and efficient ways. Additionally, these systems demonstrate remarkable adaptability, allowing them to adjust strategies based on new information or changing environments, which enhances their resilience and effectiveness in dynamic scenarios. Their capacity for workflow optimization ensures the efficient execution of multistep, complex processes. Moreover, they continuously improve by learning from historical data and feedback, further refining their performance over time. Finally, their ability to seamlessly integrate with diverse enterprise systems makes them invaluable assets in modern organizational ecosystems.
Difference between Agentic AI and other GenAI models
Generative AI and agentic AI are two distinctive stems of artificial intelligence, each with its own unique strengths and applications.
GenAI shines out at creating new content across various formats, including text, images, music, and even code. It’s adept at brainstorming ideas, crafting compelling narratives, and generating innovative solutions. Thus, generative AI primarily focuses on creation, relying on human input and advice to determine the context and goals of its output.
On the other hand, agentic AI is action-oriented. These tools can analyze situations, frame strategies, and execute actions to achieve specific goals, all with minimal to NIL human intervention. They are specifically designed to operate independently, adapt to changing environments and to learn from their experiences.
In terms of usage, generative AI is more appropriate for creative industries, where the generation of content like art, music, or text is required. Whereas, agentic AI fits in environments where autonomy and decision-making are crucial, such as robotics, autonomous vehicles, and personal assistants. However, these two tools can be used in tandem to create impactful solutions that can combine creativity with action. For example, a GenAI model can be used to create a good marketing copy, while an agentic AI tool can be utilized to autonomously deploy that copy to the optimal channels based on real-time data and campaign objectives.
Real-world applications of agentic AI
This advancement in agentic AI is already reshaping various industries, from business to healthcare, by enhancing efficiency, precision, and decision-making.
Autonomous vehicles
Agentic AI plays a crucial role in autonomous vehicles. It enables cars in navigating roads, making decisions, and adapting to changing conditions with minimal human input. With the help of sensor data and complex algorithms, these systems can assess traffic patterns, avoid obstacles, and even select optimal routes in real-time.
- Advantage: Lesser accidents, better traffic flow, better driver convenience.
- Ex: Tesla’s Autopilot and Waymo’s autonomous taxis.
Healthcare diagnostics
Agentic AI systems, equipped with advanced algorithms, can analyze medical images with unprecedented accuracy, detecting anomalies the human eye may miss. Moreover, by analyzing patient data, such as genetic information, medical history, and lifestyle factors, agentic AI can tailor treatment plans to individual patients.
- Advantage: Early diagnosis, saves time, offers personalized treatment plans.
- For example, in detecting conditions like cancer, AI-powered imaging tools can identify tumors at early stages, enabling timely interventions.
Manufacturing
Agentic AI is revolutionizing the manufacturing industry in significant ways. By optimizing workflows and minimizing waste, manufacturers can significantly lower operational costs. Real-time data analysis and management help monitor manufacturing processes and guide product quality to reduce defective products. With predictive capabilities, it further helps manufacturers accurately forecast demand, revise inventory management, and ensure timely deliveries. Additionally, Agentic AI supports sustainability efforts by reducing waste and energy consumption, contributing to a more environmentally responsible industry.
- Advantage: Less downtime, lesser maintenance costs, and high-quality products.
- Ex: AI-driven quality inspection in automotive and predictive maintenance in factories.
Financial trading
In finance, agentic AI can autonomously make trading decisions, analyze large datasets, and adjust strategies based on market trends. This helps institutions respond rapidly to changes and capitalize on opportunities with minimal human oversight.
- Advantage: Increased trading speed, reduced risk, more data-driven strategies.
- Ex: Algorithmic trading platforms, real-time portfolio management.
Business automation
In business settings, agentic AI automates complex workflows and decision-making processes. For example, in customer service, AI-driven virtual agents can handle routine inquiries, process complex transactions, and provide personalized responses by learning from interactions, reducing the need for human support.
- Advantage: Cost reduction, quicker response times, optimized resource allocation.
- Ex: Automated helpdesk responses, supply chain optimization tools
Best practices for CTOs planning to implement agentic AI
- Begin with a small project and gradually expand the adoption of AI.
- Prioritize data quality, governance, and security within the organization.
- Encourage data-driven decision-making and experimentation.
- Involve humans in critical decision-making processes.
- Collaborate with AI partners and experts to gain insights and guidance
- Develop and adhere to ethical guidelines to mitigate biases and ensure responsible AI development.
- Continuously assess the performance of AI systems to make necessary changes and adjustments.
- Establish clear roles and responsibilities among team members.
- Encourage team members to adapt, upskill, and stay updated with the latest AI trends.
Future of agentic AI
The market for AI agents is expected to grow from $5.1 billion today to a $47.1 billion market by 2030, indicating a meteoric rise ahead.
While agentic AI offers tremendous potential, several challenges and considerations must be considered. Agentic AI’s autonomous nature raises ethical questions about responsibility, accountability, and the potential for misuse. Similarly, Agentic AI systems often handle large amounts of sensitive data, making data privacy, bias, and security a critical concern. If the training data is biased, the agentic AI system may perpetuate those biases, leading to discriminatory outcomes. Ensuring AI agents can learn and improve over time without developing undesirable behaviors is very important.
In the longer term, perhaps a decade from now, the landscape of agentic AI could be dramatically different. We might see fully autonomous AI agents capable of running almost all kinds of businesses from top to bottom across all segments. The seamless integration of agentic AI with upcoming technologies could open up new possibilities. Moreover, as the emotional intelligence of AI advances, these systems might even be capable of handling complex human interactions and negotiations. This could potentially lead to reshaping a whole new world.
However, it’s important to remember that the future of agentic analytics isn’t just about smarter algorithms or more efficient data processing. It’s about fostering a symbiotic relationship between human creativity and machine intelligence (keeping humans in the loop). The most successful organizations will be those that can seamlessly continue to blend human minds with AI-driven insights, creating a new kind of collective intelligence.
In brief
Agentic AI is trending because of its ability to take action autonomously. Its ability to learn, adapt, and make smart choices is ought to create new possibilities for innovation and applications in various industries in the future.