Article
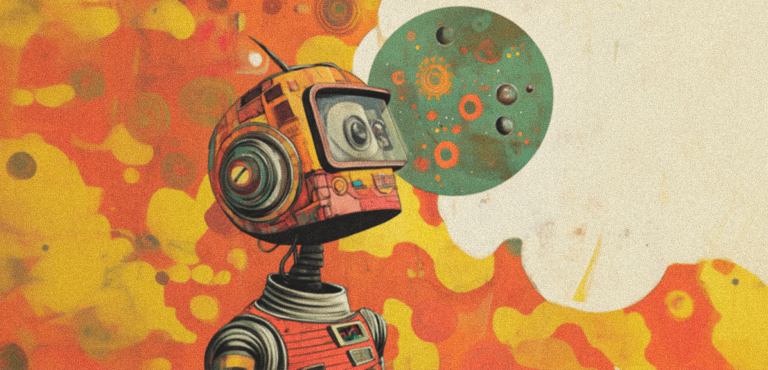
What CTOs Should Know About Generative AI Hallucinations
One intriguing and potentially disruptive phenomenon in the world of genIA are AI hallucinations: outputs from a model that seem plausible but have no basis in reality. This term draws a loose analogy with human psychology, where hallucination typically involves false perceptions.
In this article, let’s understand what the term ‘AI hallucination’ really means, how they occur, and what steps can be taken to address this concern.
What are AI hallucinations?
AI hallucinations are incorrect or misleading results that AI models generate. AI models are generally trained on data – as a result, they learn to make predictions by finding patterns in the data. However, the accuracy of these predictions often depends on the quality and completeness of the training data. If the training or fed data is incomplete, biased, or otherwise flawed, the AI model may learn incorrect patterns, leading to inaccurate predictions or hallucinations.
One infamous example of an AI hallucination happened when Google’s chatbot, Bard, made an untrue claim about the James Webb Space Telescope.
When prompted, “What new discoveries from the James Webb Space Telescope can I tell my 9-year-old about?” Bard responded with the claim that the James Webb Space Telescope took the very first pictures of the exoplanet outside this solar system. However, this information was false. In reality, the first images of an exoplanet were taken in 2004, according to NASA, and the James Webb Space Telescope was not launched until 2021. Bard’s answer was proven false by some fact-checking.
AI hallucinations are a growing concern
AI hallucinations are part of a growing list of ethical concerns about AI. Aside from misleading people with factually inaccurate information and eroding user trust, hallucinations can perpetuate biases or cause other harmful consequences if taken at face value.
For organizations, the consequences of acting on hallucinated information can be severe. Inaccurate outputs can lead to flawed decisions, financial losses, and damage to a company’s reputation.
AI systems, despite their function, are not conscious. They do not have their own perception of the world. So if you remove a human from an AI related process or if the human places its responsibility on the AI, who is going to be accountable or liable for the mistakes? This aspect is a huge point of concern.
What leaders can do to mitigate AI hallucinations?
Understanding the potential causes of AI hallucinations is important for tech leaders who are managing or working with AI models. While the tech team may not be able to eliminate AI hallucinations completely, there are concrete steps leaders can take to make hallucinations and other inaccuracies less likely.
Improve the quality of training data
High-quality and diverse data is crucial when trying to prevent AI hallucinations. If the training data is biased, incomplete, or lacks sufficient variety or information, the model will struggle to generate accurate outputs when faced with novel or edge cases. Tech leaders and developers should ensure they have more relevant and better data.
Set limitations on the number of outcomes
When training an AI model, it is important to limit the number of possible outcomes that the model can predict. Leaders and the tech team together, can constrain the result set to a smaller number and instruct the AI tool to focus on the most promising and coherent responses, which will reduce the chances of the AI model responding with inconsistent, inaccurate, or far-fetched outcomes.
Test and validate
Leaders and developers must test and validate the AI tools to ensure reliability. This process will improve the AI system’s overall performance and enable the tech team to adjust and/or retrain the model, as data ages and evolves.
Create templates for structured outputs
Leaders, with the help of developers, can create a template that tells/guides the AI models on the precise format or structure in which the information needs to be presented.
For example, if the team is training an AI model to write text, a template that includes the following elements can be prove to be helpful.
- A title
- An introduction
- A body
- A conclusion
Follow ethical AI guidelines
Leaders need to develop and adhere to ethical guidelines for the use of AI, emphasizing responsible and fair AI practices. They need to establish an AI governance framework that includes ethical considerations, ensuring alignment with organizational values.
Rely on human oversight
Leaders should integrate human oversight into critical decision-making processes involving AI. They should establish clear roles for human reviewers in order to interpret and validate AI-generated outputs. Moreover, there needs to be a continuous collaboration between AI systems and human experts to enhance decision accuracy.
Starting on a path against AI hallucinations in the future
The rate of hallucinations will decrease, but it is never going to disappear — just as even highly educated people can give out false information. As more AI models are built, new hallucination types will appear – wherein tech leaders will find themselves racing to fix and resolve the latest ones.
Continuous refinement, training, and exposure to high-quality, structured data will be necessary to ensure artificial excellence. The collective thought to shape a future where AI solutions are not just nearly perfect but are trusted, reliable, and an integral part of solving some of the world’s most pressing challenges – will still be an ongoing process for many years to come.
In brief
Despite how far we have come in terms of technology, AI still has a long way to go before it can be considered a reliable replacement for humans in a lot of tasks. However, overcoming hurdles like AI hallucinations will open up a world of possibilities that will enable us to realize the full promise of this type of emerging technology.